Terminology
- Latency: time spent waiting to be serviced
- Overloaded term (sometimes it can refer to response time)
- Response time: time for an operation to complete (waiting time/latency + service time)
- Saturation: amt/degree of queued work
- Bottleneck: limiting factor (like reagent) for the system
- Workload: Input to the system or load applied, jobs
- Cache: Faster storage area tier
PA: Analysis –> detect bottleneck –> find bottleneck –> solve bottleneck –> analysis
Time scales
Utilization:
- Time based: E[time server was busy]
- $U = \frac{B}{T}$; U = utilization; B = total time system busy over T the observation period
- Capacity based: System/component’s ability to deliver amount of throughput
- Proportion of system/component’s resources currently working
- @100% capacity-based utilization; saturation has been reached. <100%: no worries
Caching
- caches are also implemented in software.
- caching algorithm may be the bottleneck.
- hit ratio = hits / (hits + misses); higher is better
- cache miss rate: misses per second; lower is better
- Resource Analysis Perspective [by sysadmin]
- Start @devices (resource level)
- Includes perf issue investigations, and capacity planning
- Demand supply
- Workload Analysis Perspective [by devs]
- Targets
- Requests (workload applied)
- Latency (response time)
- Completion (error rate)
- Metrics
- Throughput
- Latency
Methodologies
No clear methodology until recently!
- Tools (OS specific application tools)
- List avail. perf tools
- For tool T, list useful metrics
- For metric M, list ways of interpretation
- USE (Utilization - Saturation - Errors)
- Resources: CPU/RAM/NIC/STORAGE/ACCELERATORS
- Some rscs cannot be fully monitored?
- Machine health
- RED (Request rate - Errors - Duration)
- Usu. cloud services/microservice. Check per svc.
- User health
- Workload characterzation
- Inputs rather than resultant perf.
- Who causes laod
- Why load called
- What are attributes of the load
- IOPS/Throughput/Direction (R/W).
- Include the variance when possible/appropriate
- How load changing over time
- Monitoring
- perfstats over time.
- For capacity planing/quantifying growth/peak usage
- Time series: Historic values (time-based patterns)
Capacity planning
- How well handling load as it scales.
- Predicting when service fails as the workload evolves
- Determine cost-effective ways to delay saturation.
- Challenges:
- usage load fluctuation
- peak usage
- cost of provisioning
Availability:
- Total system uptime/Total observation period x 100%
- On-premise uptime availability stndard: 99.999%
- Service Outages: Opposite of availability
This is only relevant for fixed amount of resources (c.f. 15 years ago?):
- Resource for peak load (waste rscs)
- Under-provision (lose potential revenue)
Nowadays we have automatic scaling.
Provisioning for resources have 3 main strategies:
- lead
- add cap in anticipation of dd
- lag
- add cap after full cap reached
- match
- Automatic scalers: increment in small units as dd increases.
Capacity of s system:
- Inverse rls of throughput and response time.
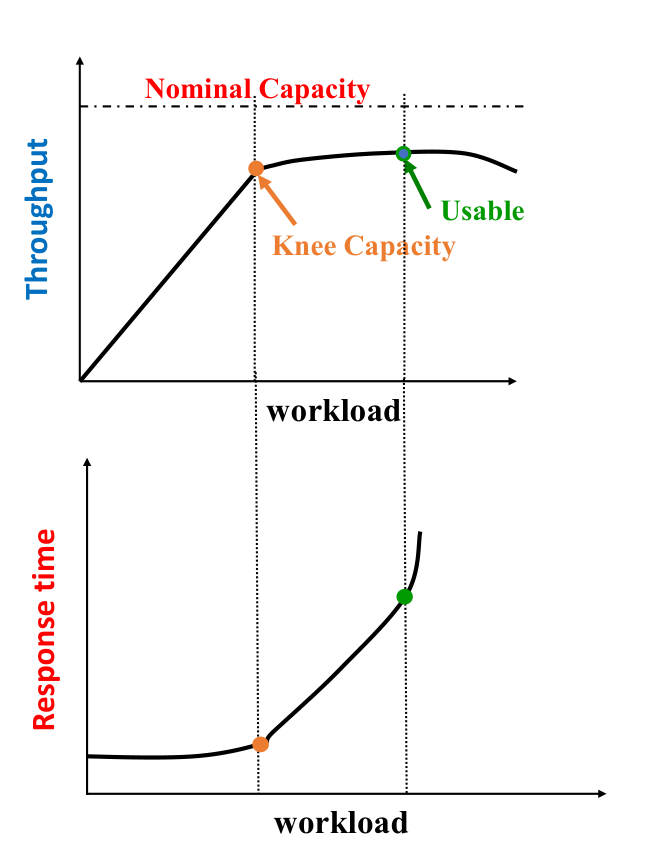
Service-Level agreements (SLA):
- determination of what app users can expect for
- response time
- throughput
- system avail
- reliability
- tie IT costs to SLA.
- if these are not met, pay fine etc.
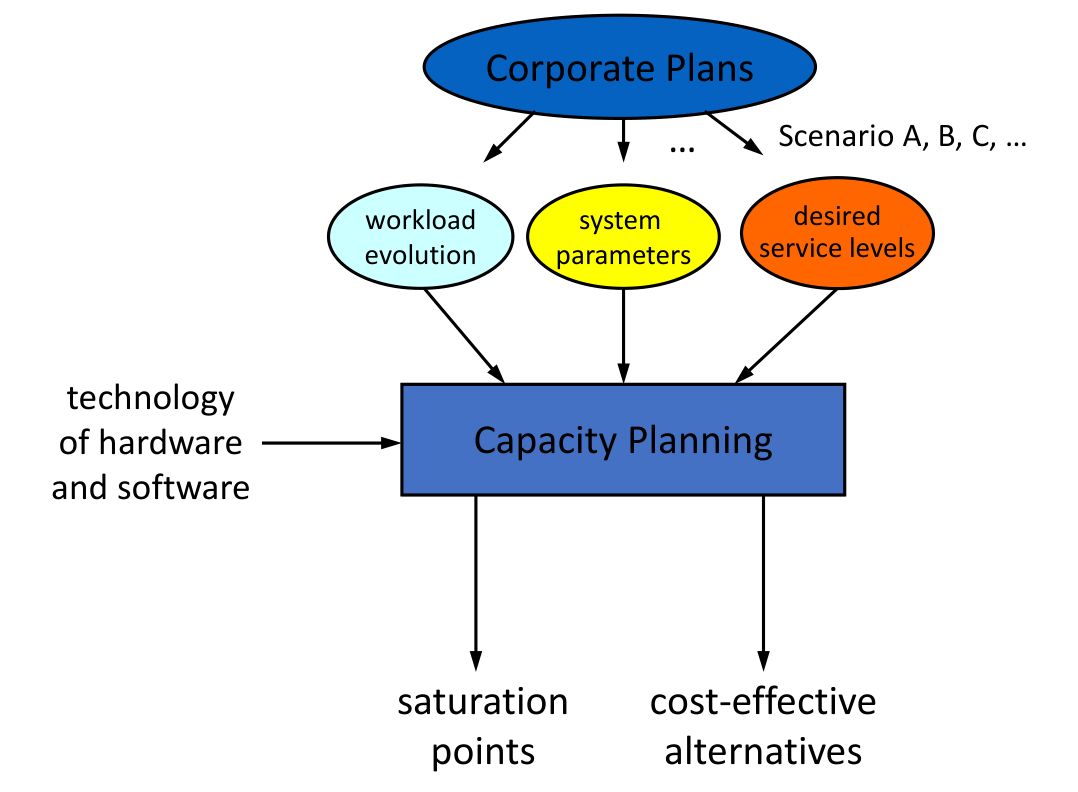
- Understand the environment: create a workload model
- Workload characterization
- Workload model validation + calibration
- Workload forecasting: Use the workload model
- Performance model development: Create a performance model
- Performance model validation + calibration
- Performance prediction: Use the performance model
- Cost Model development: Use the workload model to create a cost model
- Cost prediction: Use the cost model
- Cost/Performance analysis
Understanding the environment
Workload characterization
Only via observation. Measurements can be taken; existing knowledge (e.g. another system).
We refine this model until validation/calibration passes.
- Workload intensity
- Service demand
- trying to find more general characteristics
- easier parameters
Data collection: How do we determine param values for basic components?
- No data collection facilities available:
- Benchmark (Synthetic workload e.g. locust.io)
- Industry practice
- Rules of thumb (ROTs)
- Some:
- All of the above and measurements
- All/Detailed:
- Use measurements only